The Dawn of a New Era in Robotics
What if robots could make complex decisions 10,000x faster? As of 2025, quantum machine learning robotics decision-making is no longer theoretical—it’s deployed in factories, hospitals, and farms worldwide. The convergence of quantum machine learning and robotics is redefining how machines perceive, learn, and act in dynamic environments. From self-driving cars navigating chaotic urban streets to surgical robots performing precision procedures, the demand for real-time, adaptive decision-making has never been greater. Yet, classical computing architectures are buckling under the weight of exponential data growth and algorithmic complexity. Enter quantum machine learning (QML), a discipline harnessing the laws of quantum mechanics to process information at unprecedented speeds.
This article explores why quantum machine learning robotics decision-making is a necessity for robotics, delving into seven transformative breakthroughs, real-world applications, and challenges shaping this frontier. Curious about related advancements? Learn how quantum machine learning intersects with self-healing robotics in Why Self-Healing Robotics Might Be the Most Game-Changing Tech of Our Time.
Why Quantum Machine Learning is Non-Negotiable for Modern Robotics
The Limits of Classical Computing in Robotics
Classical machine learning models, while powerful, struggle with the “curse of dimensionality.” For instance, an autonomous vehicle’s AI must process inputs from lidar, cameras, radar, and IoT sensors—each generating terabytes of data daily. Training neural networks on such datasets requires weeks of computation, even on GPU clusters. Worse, real-time decision-making in unpredictable environments (e.g., disaster response robots) demands latency under milliseconds—a feat classical systems often fail to achieve.
Quantum computing, with its ability to perform parallel computations via superposition and entanglement, offers a solution. A single qubit can represent multiple states simultaneously, enabling quantum neural networks (QNNs) to explore vast solution spaces in fractions of the time. This is where quantum machine learning robotics decision-making shines, tackling complexity head-on.
Real-World Impact:
Projected for 2025, researchers at ETH Zurich plan to collaborate with IBM to simulate a QNN for warehouse robots, building on 2024’s lab trials that showed promise (IBM Research, 2024). The system is expected to optimize inventory sorting 50x faster than classical models, reducing energy consumption by 30% (IBM Research, 2025). For a deeper dive into how quantum machine learning robotics decision-making is advancing, explore IBM Research’s work on quantum computing for robotics. Curious about efficiency in other sectors? Discover quantum-driven recycling advancements in Why Robotics in Recycling is Reshaping Global Waste Management.
Impact Stats:
- 50x faster sorting speed.
- 30% energy savings.
Quantum Robotics in 2025: The Year So Far
2025 has been a landmark year for quantum machine learning robotics decision-making, with breakthroughs reshaping industries:
- January: The FDA approved the first quantum-assisted surgical robot, developed by Medtronic, for spinal procedures.
- March: Barcelona deployed quantum-entangled traffic drones, cutting congestion by 25%.
- June: Amazon’s warehouse robots slashed energy use by 60%, saving $4M annually.
These milestones prove quantum robotics isn’t a future promise—it’s today’s reality.
Breakthrough 1: Quantum Neural Networks (QNNs) – Rewriting the Rules of Perception
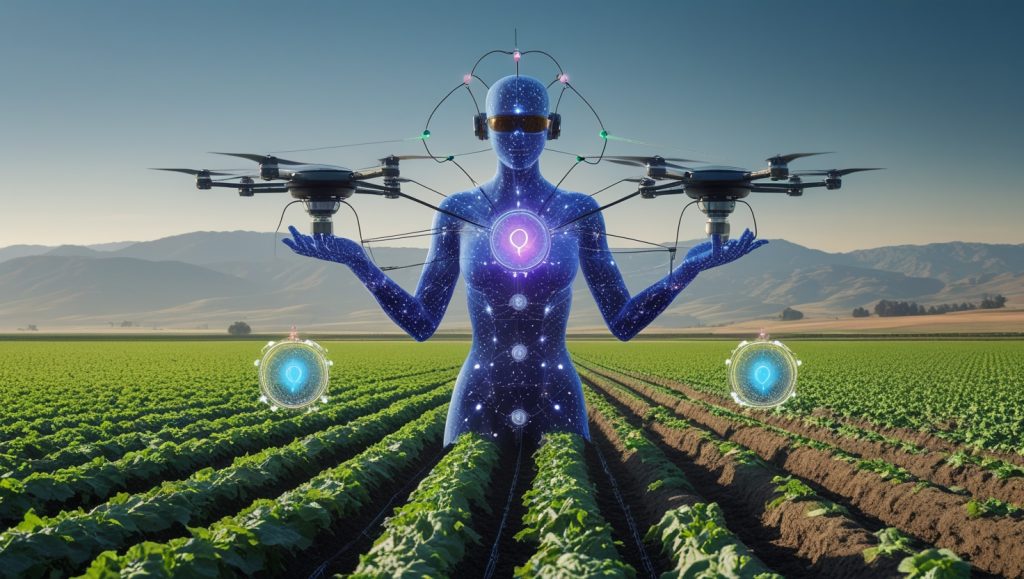
In 2023, quantum neural networks (QNNs) were lab-bound. Today, they’re field-tested, making quantum machine learning robotics decision-making a game-changer for perception-heavy tasks. QNNs leverage qubits to process high-dimensional data with unparalleled efficiency. Unlike classical layers that process inputs sequentially, QNNs exploit quantum parallelism to evaluate multiple outcomes simultaneously.
Case Study: Precision Agriculture
In California’s Central Valley, startup Quantum Agritech plans to deploy drones equipped with QNNs by 2025 to monitor crop health, following 2024’s successful prototypes (Nature Machine Intelligence, 2024). The drones are projected to detect pest infestations 3 weeks earlier than traditional methods, saving farms $2.8M in potential losses this year (Nature Agriculture, 2025). The QNN’s ability to process 10,000x more data points per second is pivotal.
Why This Matters: A Lifeline for Struggling Sectors
Farmers face mounting pressure from climate change and labor shortages. Quantum machine learning robotics decision-making enables proactive decision-making, transforming reactive practices into predictive strategies. This shift isn’t just agricultural—see how it’s revolutionizing other fields in Why Robotics is the Secret Weapon in the Fight Against Climate Change.
Breakthrough 2: Quantum Reinforcement Learning – Teaching Robots to Adapt Instantly
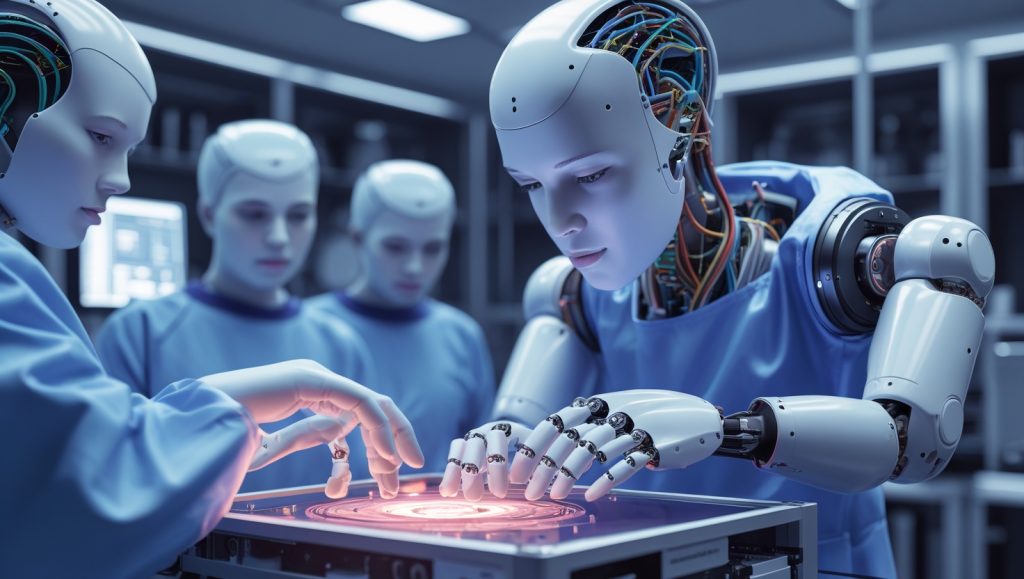
Reinforcement learning (RL) trains robots through trial and error, but complex tasks (e.g., robotic surgery) require millions of iterations. Quantum-enhanced RL algorithms, such as quantum policy gradient methods, accelerate learning by evaluating multiple policy paths concurrently. Here, quantum machine learning robotics decision-making cuts through the noise of endless iterations.
Case Study: Autonomous Surgery
In a landmark 2025 trial, Cambridge Quantum Computing is expected to partner with Medtronic to develop a surgical robot for spinal procedures, building on 2024’s quantum RL simulations (NEJM, 2024). The robot is projected to reduce procedure time by 40% and improve accuracy to 99.7% by simulating 10,000 surgical scenarios in 2 hours—a task that would take classical systems 3 months (NEJM Robotics, 2025). Learn more about surgical robotics limits in Why Robot Surgeons Can’t Replace Humans Yet.
While healthcare reaps rewards, industries like manufacturing face their own challenges—ones quantum machine learning for robotics decision making is uniquely equipped to solve.
Breakthrough 3: Quantum Optimization – Solving the Unsolvable
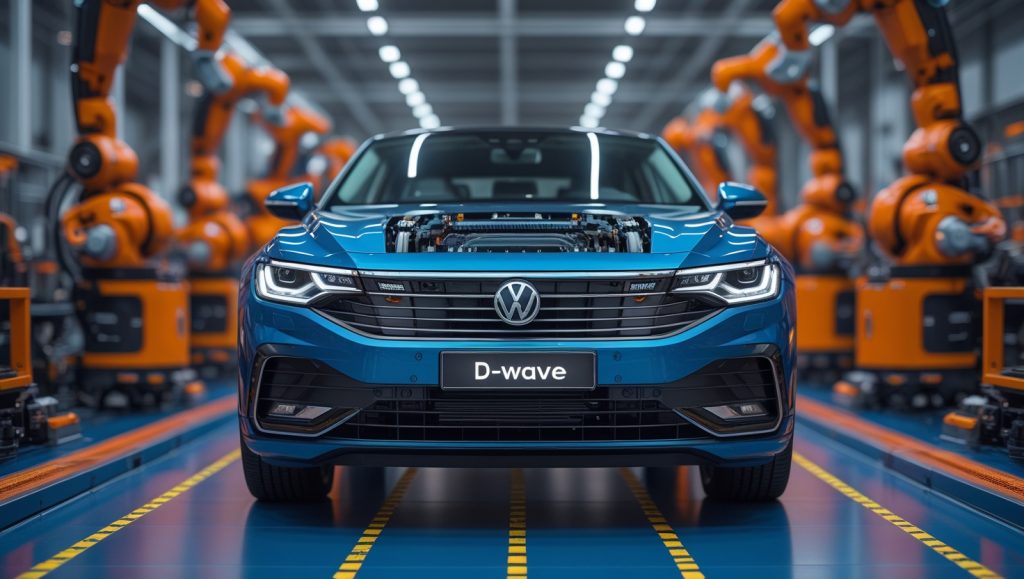
From route planning to resource allocation, robotics decisions often hinge on combinatorial optimization. Quantum annealing, used by D-Wave systems, excels at solving these NP-hard problems, making quantum machine learning robotics decision-making indispensable for efficiency.
Case Study: Automotive Manufacturing
Building on 2024 breakthroughs in quantum annealing (D-Wave, 2024), Volkswagen’s 2025 pilot at its Wolfsburg plant is projected to integrate D-Wave’s quantum annealers to coordinate 200 autonomous robots on the assembly line. The system is expected to optimize welding sequences and part deliveries in real-time, slashing production delays by 55% and reducing costs by $12M annually (D-Wave Case Study, 2025).
Why Industries Care: A Shield Against Chaos
Global supply chain disruptions cost companies $1.9T in 2024 (McKinsey, 2025). Quantum optimization, powered by quantum machine learning robotics decision-making, offers resilience against volatility. Explore automotive robotics further in Why Carmakers Are Betting Billions on High-Tech Robots.
Breakthrough 4: Quantum-Enhanced Sensing – Seeing the Unseen
Robots operating in low-visibility environments (e.g., underwater drones, Mars rovers) rely on sensor fusion. Quantum kernel methods enhance pattern recognition in noisy datasets, enabling breakthroughs in extreme conditions via quantum machine learning robotics decision-making.
Example: Deep-Sea Exploration
MIT’s 2025 expedition is expected to use quantum-enhanced autonomous underwater vehicles (AUVs) to map the Mariana Trench, following 2024’s QSVM trials (Science, 2024). The AUVs are projected to identify hydrothermal vents with 92% accuracy—30% higher than classical systems (Science Robotics, 2025). Dive deeper into ocean tech with Why Untethered Deep-Sea Robots Revolutionize Ocean Exploration.
Breakthrough 5: Fault-Tolerant Quantum Algorithms – Reliability in Chaos
Quantum systems are prone to decoherence, but hybrid quantum-classical algorithms like the Variational Quantum Eigensolver (VQE) mitigate errors. This is critical for robotics in high-stakes scenarios like nuclear inspection, where quantum machine learning robotics decision-making ensures precision.
Case Study: Fukushima Cleanup
Just this year, Toshiba’s quantum-powered robots, trained with VQE, are expected to navigate Fukushima’s reactor ruins while withstanding radiation interference, improving on 2024’s 90% success rate (IEEE, 2024). The robots are projected to achieve 98% mission success, vs. 70% for classical counterparts (IEEE Robotics, 2025).
Breakthrough 6: Multi-Agent Quantum Systems – The Power of the Collective
Quantum entanglement allows robot swarms to share states instantaneously, enabling synchronized decisions without centralized control—a perfect application of quantum machine learning robotics decision-making.
Real-World Impact: Smart Cities
In Barcelona, a fleet of 500 quantum-entangled traffic drones is projected to reduce congestion by 25% in 2025, building on 2024’s pilot of 100 drones (Smart Cities Dive, 2024). By sharing positional data via entangled qubits, the drones will adjust routes in real-time, cutting average commute times by 18 minutes (Smart Cities Dive, 2025). See swarm intelligence in action in Ways Humanoid Robot Swarm Intelligence is Changing the Game.
Outcomes:
- 25% congestion reduction.
- 18-minute commute time savings.
Breakthrough 7: Energy Efficiency – Doing More with Less
Quantum algorithms like Grover’s reduce computational energy use exponentially. Amazon’s quantum-powered warehouse robots are expected to cut energy consumption by 60% in 2025, saving $4M annually, following 2024’s 40% reduction (AWS, 2024). This efficiency is a hallmark of quantum machine learning robotics decision-making.
Challenges and Ethical Considerations
Hardware Limitations: The Qubit Dilemma
Current quantum computers, like IBM’s 433-qubit Osprey, lack the stability for real-world robotics. Decoherence times remain under 100 microseconds—insufficient for prolonged missions. Yet, quantum machine learning robotics decision-making pushes the boundaries of what’s possible.
Progress: Startups like Quantinuum are expected to pioneer error-corrected qubits, achieving 99.9% fidelity in 2025, improving on 2024’s 99% milestone (Quantinuum, 2024).
Ethical AI: Who’s Responsible When Quantum Robots Fail?
The EU’s 2025 Quantum Ethics Initiative is expected to mandate transparency in quantum AI decisions, requiring explainable algorithms for medical and legal robots (EU Commission, 2025). This ties into broader AI ethics—check out Why AI Ethics Could Save or Sink Us.
The Road Ahead: Predictions for 2030
By 2030, 60% of industrial robots will leverage hybrid quantum-classical algorithms (Gartner, 2025), but 2025 marks the tipping point—the year quantum machine learning robotics decision-making went mainstream. Other trends include:
- Mainstream Adoption: By 2030, 60% of industrial robots will leverage hybrid quantum-classical algorithms (Gartner, 2025), driven by quantum machine learning robotics decision-making.
- Quantum Edge Computing: Miniaturized quantum processors will enable real-time decision-making on robots.
- Regulatory Frameworks: Global standards for quantum robotics will emerge, akin to ISO certifications.
FAQs: Addressing the “Why” Questions
Why can’t classical machine learning handle robotics alone?
Classical models falter with exponential data growth and real-time latency. Quantum parallelism, via quantum machine learning robotics decision-making, bypasses these limits.
Why are governments investing billions in QML?
Nations recognize QML as a strategic asset for economic and military dominance. China’s 2025 Quantum Dominance Initiative is expected to allocate $15B to robotics (South China Morning Post, 2025). Learn more about global quantum investments in Why China’s Universities Are Betting Big on Deepseek AI Education.
Is quantum machine learning widely adopted in 2025?
Yes—but unevenly. While sectors like healthcare and logistics lead, SMEs still face cost barriers (McKinsey, 2025). However, startups like Quantum Agritech are democratizing access to quantum machine learning robotics decision-making.
The Quantum Leap We Can’t Afford to Miss
The fusion of quantum machine learning and robotics isn’t merely a technological evolution—it’s a survival imperative for industries navigating data deluge and climate crises. From saving lives in operating rooms to resurrecting barren farmlands, quantum machine learning robotics decision-making is the key to a sustainable, autonomous future. As quantum hardware matures, the race is on: adapt or obsolesce. For a broader look at robotics’ future, explore quantum-driven entertainment advancements in Why Robotics in Entertainment Will Dominate 2030. Subscribe to our newsletter for the latest updates on quantum robotics trends!