The Coffee-Spilling Reality Check
In March 2025, Stanford’s Robotic Interaction Lab hosted a demo of their newest AI-powered robot, designed to serve coffee to researchers. Despite training on 10,000 simulated hours of perfect pours, the bot spilled scalding coffee onto a grad student’s laptop when faced with a slightly tilted mug. The incident went viral, symbolizing a harsh truth: AI in robotics excels in controlled labs but falters in the messy unpredictability of reality. While ChatGPT writes poetry and DeepMind solves protein folding, robots still struggle with tasks toddlers master—like holding a cup. Why? And what will it take to bridge this gap?
1. Why Text Prediction Doesn’t Translate to Physical Intelligence
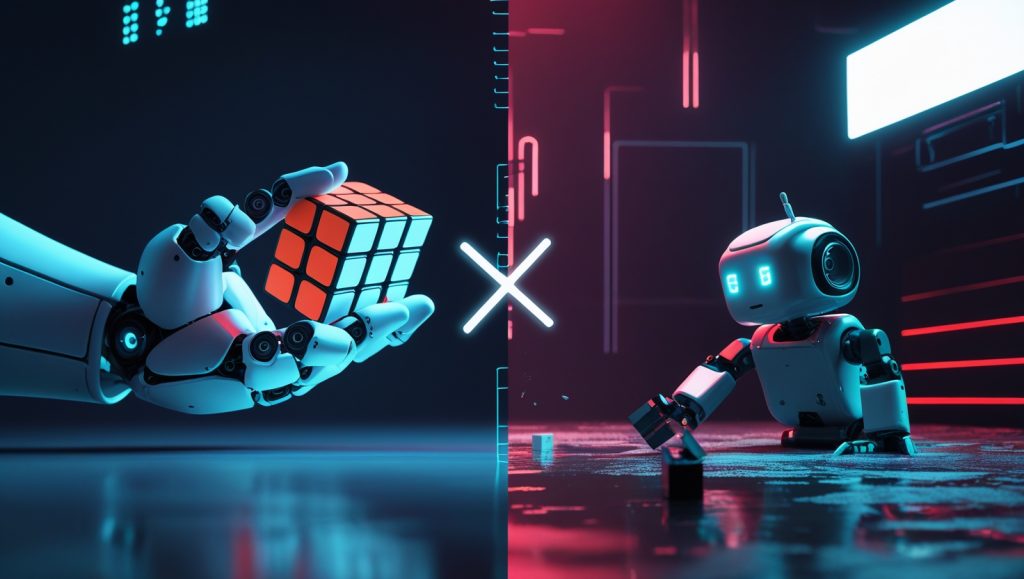
Language models like GPT-6 thrive on static datasets, but robots operate in dynamic environments where milliseconds and millimeters matter. Three fundamental disparities explain this chasm:
The Simulation-Reality Gap
AI models train in pristine digital worlds, but robots face real-world noise—slippery floors, glare from sunlight, or a stray LEGO block. In 2025, MIT’s Rodney Brooks revealed that 90% of robotic “breakthroughs” fail outside labs due to overfitting to idealized conditions. For example, OpenAI’s Dactyl robotic hand solved a Rubik’s Cube in simulation in 2020 but took 18 months to replicate it physically—and only with hardware8172 tweaks costing $2 million.
Latency: The Silent Killer
Human reflexes operate at 150ms; Boston Dynamics’ Atlas processes sensor data in 500ms. This lag is catastrophic for tasks like autonomous driving, where a 0.3-second delay can be fatal. While NVIDIA’s Jetson Orin edge AI chips aim to cut latency to 200ms by 2026, true real-time response remains a mirage. For deeper insight into how AI in robotics tackles such challenges, check out Why Self-Driving Cars Keep Crashing, which unpacks the hidden flaws stalling autonomous tech.
The “Common Sense” Deficit
LLMs infer context from text, but robots lack innate physics understanding. When UC Berkeley researchers instructed a robot to “put the cooled soup in the microwave,” it froze—unable to reconcile cooling with reheating. Google’s RT-2 model attempts to merge language and action, but its success rate in novel tasks is just 32%. This gap in reasoning is a persistent thorn in the side of AI in robotics, revealing how far we are from true adaptability. A recent NPR report from March 31, 2025, AI and ChatGPT: Software Meets Robotic Tasks, highlights similar struggles, noting that even cutting-edge language models falter when tasked with guiding robots through real-world scenarios—further proof that text prediction doesn’t easily translate to physical smarts.
2. Why AI in Robotics Is Succeeding in Structured Environments
Despite chaos in open-world settings, AI in robotics thrives where variables are controlled:
Autonomous Mobile Robots (AMRs): Warehousing’s Quiet Revolution
In 2025, DHL deployed 5,000 AI-driven AMRs across U.S. warehouses. These bots use LiDAR and reinforcement learning to navigate around fallen pallets and human workers, reducing picking errors by 70%. The key? Warehouses are predictable grids with fixed layouts. As DHL’s CTO noted: “Our floors are mapped to the millimeter. The real world isn’t this tidy.” For more on how AI in robotics transforms labor-intensive sectors, see Why Robots Solve the Labor Crisis, which dives into the promise and pitfalls of robotic workforce solutions.
Surgical Robotics: Precision Over Instinct
Intuitive Surgical’s Da Vinci 5 uses AI to filter out a surgeon’s hand tremors with 0.01mm precision. Its success lies in structured workflows: surgeries follow repetitive steps where AI excels. In 2025, the bot performed 1.2 million procedures globally, with complication rates 40% lower than human-only surgeries. This precision hints at a broader trend in AI in robotics, where controlled environments amplify its strengths—though it’s worth noting Why Robot Surgeons Can’t Replace Humans Yet for a fearless look at the limits still holding it back.
Industrial Cobots: Scripted Excellence
Tesla’s Optimus robots now install car seats in Fremont factories, using imitation learning to mimic human workers’ motions. While they can’t improvise, their error rate in scripted tasks is 0.2%. “They’re brilliant at repetition, terrible at surprises,” admitted Tesla’s VP of Robotics.
3. Why AI in Robotics Fails at the “Last 1%”
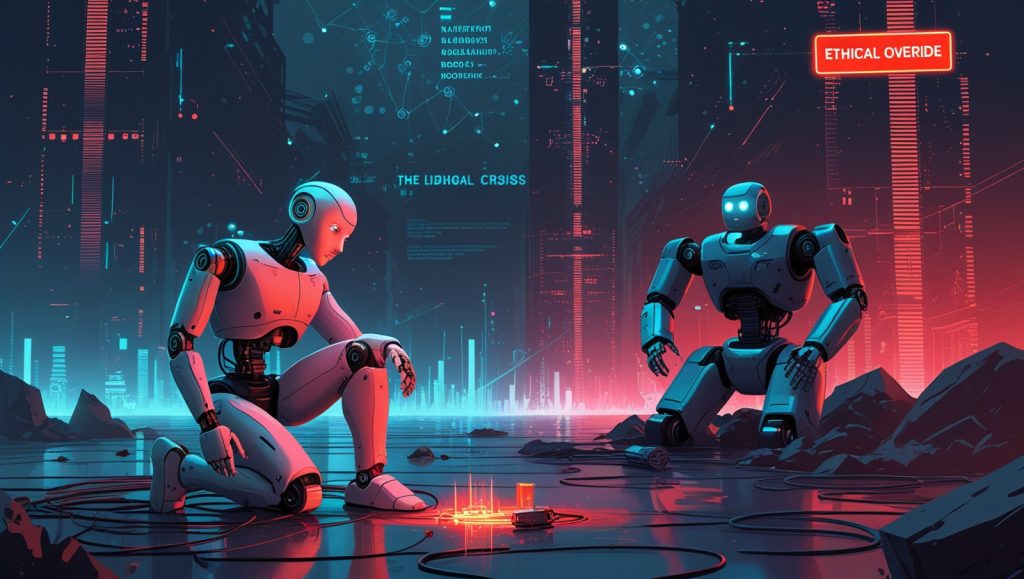
Rodney Brooks’ 2025 Robotics Scorecard highlights five unyielding barriers:
The Three-Legged Stool Problem
Robotics requires advances in hardware, software, and energy. While AI progresses, battery tech lags: Agility’s Digit humanoid drains 3kW/hour, limiting runtime to 90 minutes. Meanwhile, actuators remain prone to wear—Boston Dynamics’ Spot requires $15,000/year in maintenance. This trifecta of challenges keeps AI in robotics from reaching its full potential, a point echoed in Why Robotics Is the Secret Weapon Against Climate Change, which explores how energy inefficiencies hinder broader impact.
Data Scarcity: The Hidden Crisis
LLMs train on trillion-token datasets, but robotic data is scarce. MIT’s Dex-Net contains just 10 million grasp attempts—a fraction of text corpora. This forces models to generalize poorly. When Toyota tried training a bot on 100,000 virtual kitchens, it failed to handle real-world cabinet handles 60% of the time. The scarcity of real-world data remains a bottleneck for AI in robotics, stunting its ability to adapt beyond labs.
The Cost Chasm
A single robotic arm costs $50k; human labor is $15/hour in India. Until prices drop 10x, adoption will remain niche. Even Amazon’s Astro home robot flopped in 2024 due to its $1,600 price tag—too steep for a gadget that couldn’t climb stairs. This economic reality keeps AI in robotics out of reach for many, a topic dissected further in Why Robot Subscription Services Are the Next Big Revenue Stream, which examines innovative cost-lowering models.
Ethical Quicksand
In Tokyo, an Amazon delivery robot blocked an ambulance in 2025, delaying critical care by 8 minutes. Unlike text errors, robotic failures have physical consequences. The EU’s AI Liability Directive now mandates “kill switches” on all public robots, but enforcement is patchy.
4. Why Hybrid Intelligence Is the Only Path Forward
The future of AI in robotics lies in collaboration, not replacement:
Human-AI “Co-Pilots”
Goldman Sachs reports factories using AI assistants (robots handle precision tasks; humans manage exceptions) achieve 40% higher productivity than full automation. At Siemens’ Berlin plant, workers use AR glasses to guide robots through custom welds, blending human intuition with machine precision. This hybrid approach is gaining traction globally—explore Why China’s Industrial Robot Dominance Is Reshaping Manufacturing for a look at how such synergies are scaling in Asia’s factories.
Synthetic Data: Training in the Matrix
NVIDIA’s Isaac Sim generates synthetic scenarios—spills on wet floors, tangled wires—to teach robots rare edge cases. BMW cut real-world training time by 80% using this method, deploying bots to handle custom car interiors in Munich. The use of simulated environments is a game-changer for AI in robotics, accelerating progress where physical data falls short.
Bio-Inspired Designs
Stanford’s FarmHand mimics octopus tentacles to grasp irregular produce. Its AI plans grasps, while soft actuators adapt to squishy tomatoes or rigid potatoes. In 2025, it reduced farm waste by 30% in California’s Central Valley. This bio-mimetic approach showcases how AI in robotics can borrow from nature to solve practical problems, a concept expanded in Soft Robotics Artificial Muscles Breakthrough.
5. Why Regulators Are Racing to Catch Up
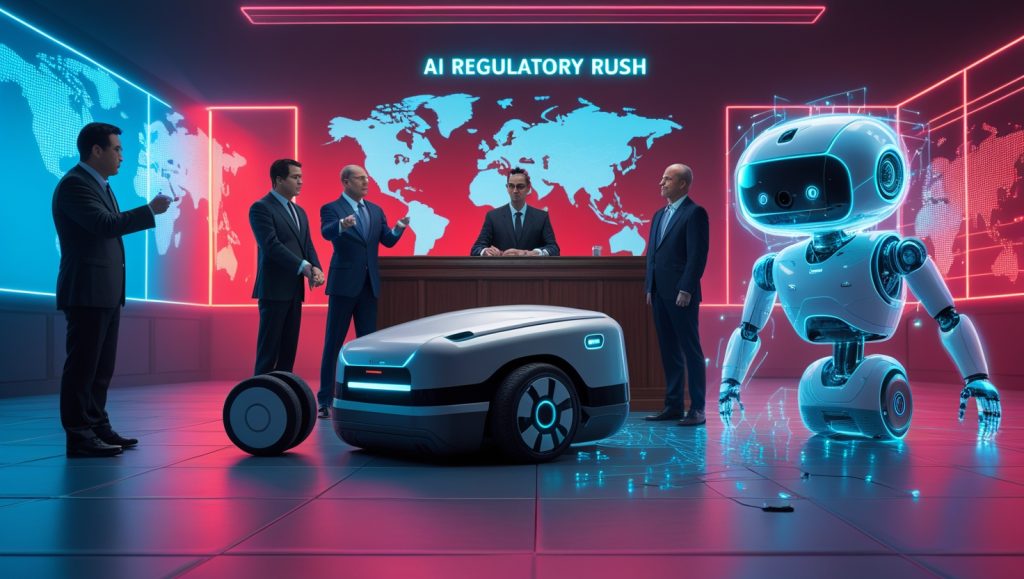
As AI in robotics advances, lawmakers grapple with unintended consequences:
The Liability Labyrinth
When a food delivery bot in San Francisco malfunctioned and caused a bike accident in 2025, courts debated who was liable: the AI developer, the operator, or the city. The U.S. Autonomous Systems Accountability Act (2026) now assigns liability to operators, but loopholes remain. This legal tangle complicates the rollout of AI in robotics, especially in public spaces.
Privacy vs. Progress
Amazon’s Astro home robot sparked outrage when internal documents revealed it shared floor plan data with advertisers. The EU’s AI Ethics Act (2026) bans such practices, but U.S. regulations lag. For a broader take, Why AI Ethics Could Save or Sink Us dives into the ethical stakes of such tech missteps.
Global Fragmentation
China’s 2025 Robotics Standards mandate backdoor access for regulators, while the EU demands local data storage. This fragmentation stifles innovation: iRobot’s CEO lamented, “We’re building 20 versions of the same bot to comply with regional laws.”
6. Why the Next Decade Will Be Make-or-Break
By 2035, AI in robotics must overcome three existential challenges:
Energy Efficiency
Current robots consume 50x more power than human equivalents. MIT’s Mini Cheetah 3 aims to slash this via liquid-cooled actuators, but prototypes remain years from commercialization. Until energy efficiency improves, AI in robotics will struggle to scale sustainably.
Generalization
Today’s bots are specialists—a forklift bot can’t sort packages. DeepMind’s RoboCat (2025) learns multiple tasks via self-supervised learning, but its success rate drops from 80% to 30% when switching domains.
Public Trust
A 2025 Pew survey found 62% of Americans distrust robots in healthcare or childcare. Building trust requires transparency: OpenAI now livestreams robot training data, but skeptics call it “ethics theater.” For more on perception challenges, see Why Humanoid Robots Creep Us Out, which tackles the uncanny valley head-on.
The Delusion of Imminence
The hype around AI in robotics obscures a truth: physical intelligence evolves slower than digital. As Brooks quips, “Robotics is the art of making the barely possible work reliably.” By 2030, we’ll have bots that fold laundry—but they’ll still need humans to untangle the socks.