What if the cure for cancer lies hidden in a sea of data too vast for human minds to navigate? In 2025, AI-Driven Scientific Discovery is turning this question into reality, powering breakthroughs at an unprecedented pace. While traditional research struggles with complexity, AI-Driven Scientific Discovery is unlocking solutions once thought decades away, from decoding protein structures to forecasting climate disasters.
This revolution, driven by AI in research breakthroughs, is redefining what science can achieve. Yet, with such power comes the need for accountability. How do we ensure AI-Driven Scientific Discovery remains ethical and equitable? Let’s explore the breakthroughs, controversies, and future of this transformative era. For example, AI-assisted artifact recovery shows how AI-Driven Scientific Discovery preserves history while tackling modern challenges.
The Crisis in Modern Research: Why AI Isn’t Optional Anymore
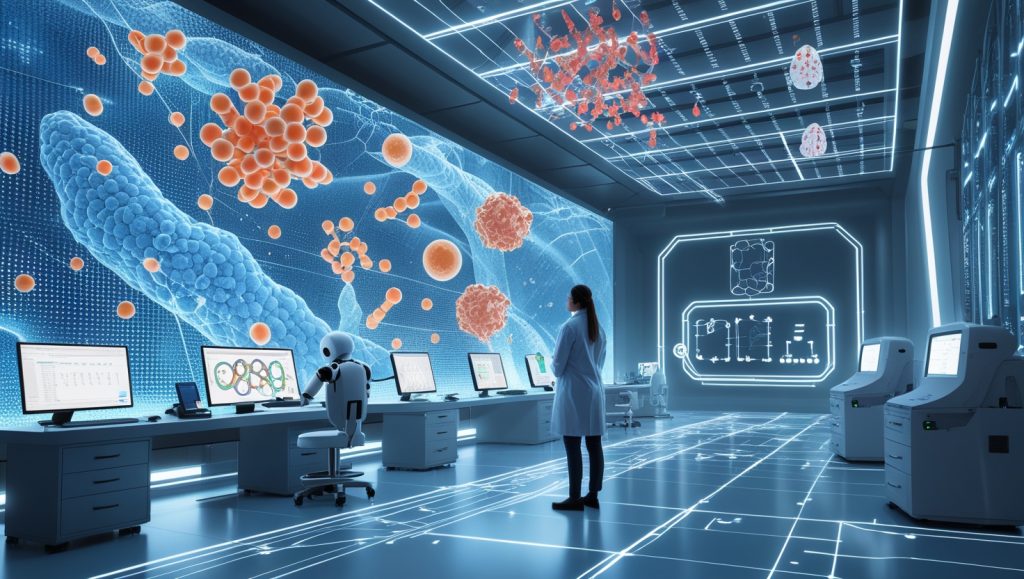
In 2021, Stanford researchers noted global scientific output doubles every 17 years. By 2025, that cycle has shrunk to 12 years. Despite this data surge, challenges like antibiotic resistance and renewable energy storage persist. The bottleneck? Human limitations in processing and connecting vast datasets, where AI-Driven Scientific Discovery becomes essential.
Data Overload: When More Isn’t Better
The Human Cell Atlas, mapping every cell in the human body, generates 10 petabytes of data yearly—equivalent to 20 million filing cabinets of text. Traditional tools falter, but AI-Driven Scientific Discovery excels, spotting patterns humans miss.
Dr. Sarah Teichmann, co-founder of the Human Cell Atlas, explains:
“AI doesn’t just handle volume—it spots patterns humans might never see. For example, our collaboration with DeepMind revealed unexpected immune cell behaviors that are now reshaping cancer immunotherapy.” This builds on advancements like AlphaFold’s groundbreaking protein structure predictions, which have accelerated biological research by decoding complex molecular data in record time.
This mirrors how quantum machine learning in robotics enhances decision-making, a parallel to AI-Driven Scientific Discovery in research.
Why AI Solves the Data Crisis
The data deluge demands tools that analyze at scale, and AI-Driven Scientific Discovery delivers by automating pattern recognition and hypothesis testing. For instance, it processes genomic sequences in hours, enabling personalized medicine breakthroughs. Smaller labs, however, face cost barriers, a challenge we’ll address later. The fearless truth: without equitable access, AI-Driven Scientific Discovery risks widening research disparities.
2025’s AI-Driven Breakthroughs: From Theory to Tangible Impact
1. Drug Discovery: From 10 Years to 10 Months
The traditional drug development pipeline costs $2.6 billion and takes over a decade. In 2025, AI-Driven Scientific Discovery slashes both numbers.
Case Study: COVID-24 Antiviral Development
When a novel coronavirus variant emerged in late 2024, Insilico Medicine’s AI platform identified a promising antiviral candidate in 46 days. By cross-referencing viral protein structures with existing compound libraries, the system prioritized molecules likely to inhibit viral replication. Human researchers then validated the top candidates, accelerating trials by 8 months.
This speed is akin to how AI in disaster response optimizes real-time crisis management, showcasing AI’s cross-disciplinary impact.
Why AI Accelerates Drug Discovery
AI’s ability to simulate molecular interactions reduces trial-and-error phases. By predicting binding affinities and toxicity risks, AI in research breakthroughs cuts costs and timelines. Yet, ethical concerns linger—can AI prioritize profitable drugs over neglected diseases? The fearless truth: without global oversight, market-driven biases could skew priorities.
2. Climate Modeling: Predicting the Unpredictable
The 2023 IPCC report warned of irreversible climate tipping points by 2030. In response, AI-Driven Scientific Discovery is now the linchpin of next-gen climate models.
Example: Google’s GraphCast
Launched in 2024, this AI model predicts weather patterns up to 10 days in advance with 50% greater accuracy than traditional methods. More crucially, it simulates decades-long climate trends in hours, helping policymakers test interventions like ocean iron fertilization or carbon capture grids.
This precision aligns with why robotics in recycling is reshaping sustainability, as both leverage AI for environmental impact.
Why AI Excels in Climate Prediction
Traditional models struggle with chaotic systems like weather, but ethical AI in science integrates diverse datasets—ocean currents, deforestation rates, emissions—to forecast with clarity. The catch? Overreliance on AI risks sidelining ground-truth data from field researchers, a gap that demands hybrid approaches.
The Ethical Tightrope: Innovation vs. Responsibility
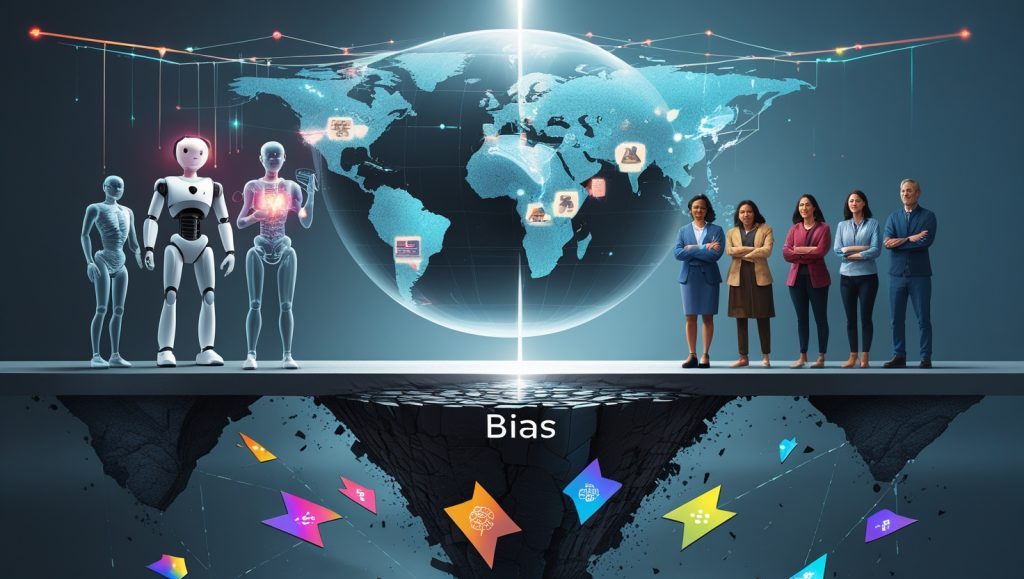
Bias in the Machine: When AI Repeats History
In 2024, a landmark study in The Lancet Digital Health revealed that AI models trained on U.S. and European medical data misdiagnosed heart disease in South Asian patients 34% more often. The culprit? Underrepresentation of diverse genetic and lifestyle data in training sets.
Dr. Priya Agrawal, bioethicist at Johns Hopkins, warns:
“AI amplifies existing biases. If we don’t intentionally build inclusivity into datasets, we risk cementing health disparities for generations.”
Solution: Initiatives like the Global AI Health Alliance now require models to be validated across at least 10 geographically diverse datasets before deployment.
This issue echoes concerns in why AI ethics could save or sink us, where systemic flaws threaten equitable outcomes.
Why Bias Persists and How to Fix It
Bias in AI stems from skewed training data and homogenous development teams. Ethical AI in science demands diversity in both datasets and creators. Programs like diversity in AI research are pushing for inclusive innovation, but progress is slow. Fearlessly, we must call out tech giants for prioritizing speed over fairness—lives depend on it.
Collaboration in the Age of AI: Breaking Down Silos
Open Science: The New Competitive Edge
In 2023, Merck and Pfizer shocked the industry by open-sourcing an AI model for Parkinson’s drug target identification. The result? A 70% increase in viable candidates, with 30% attributed to external researcher contributions.
Dr. Michael Lin, Merck’s Head of AI Innovation, reflects:
“The old ‘lab vs. lab’ mentality is obsolete. Today, sharing AI tools multiplies our collective impact. It’s not charity—it’s smart science.”
This shift mirrors open-source AI in pharma, where collaboration drives breakthroughs.
Why Open Science Fuels AI Progress
Open-source AI democratizes access, allowing smaller labs to leverage tools like AI model distillation for cost-effective innovation. However, corporate hesitancy to share proprietary models stifles progress. The bold reality: hoarding AI tools risks delaying solutions to global crises.
Public Trust: The Make-or-Break Factor
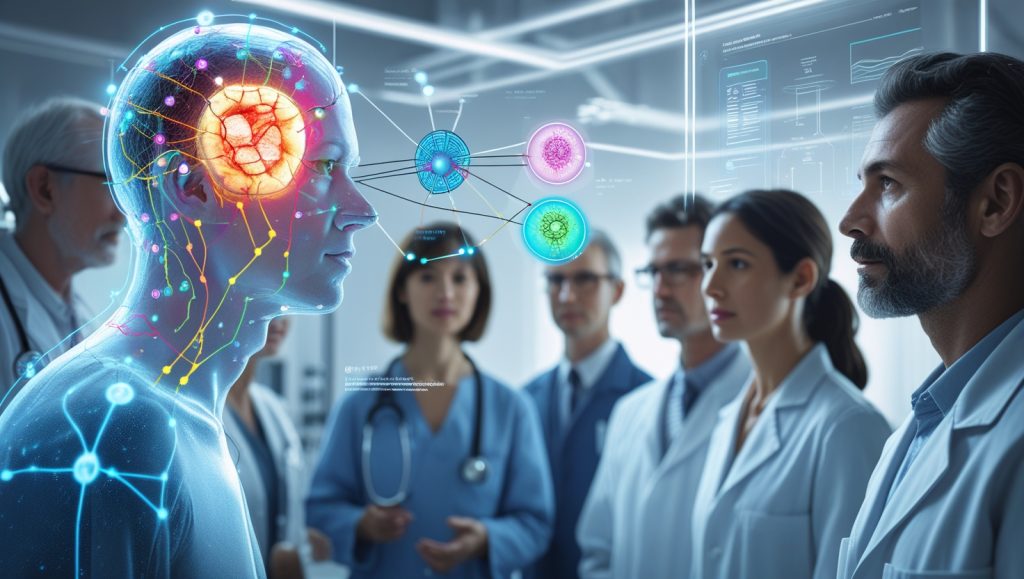
The “Black Box” Problem
A 2025 Pew Research study found that 62% of adults distrust AI-generated medical recommendations unless accompanied by plain-language explanations. Enter explainable AI (XAI)—systems that “show their work” like a student solving a math problem.
Example: DARPA’s Explainable AI Program
This program creates AI that visually maps decision pathways, like highlighting lesion features in skin cancer diagnoses, building trust in AI-Driven Scientific Discovery.
This transparency is critical, as seen in why explainable AI is the future, ensuring trust in high-stakes applications.
Why Trust Hinges on Transparency
Opaque AI erodes confidence, especially in medicine. AI-powered scientific innovation must embrace XAI to close the trust gap. Without it, skepticism could halt adoption, delaying life-saving discoveries from AI-Driven Scientific Discovery.
FAQ: Addressing the Elephant in the Lab
Will AI replace scientists?
No—it’s augmenting them. A 2025 MIT study found AI tools save researchers 13 hours/week on data processing, freeing them for creative tasks like hypothesis generation and experimental design.
Can small labs afford AI tools?
Yes. Cloud-based platforms like AWS AI Lab and Google BioML offer pay-as-you-go access to supercomputing power. Grants from organizations like CZI Meta’s Open Science Fund also subsidize costs.
How reliable are AI discoveries?
Peer review remains crucial. The FDA now mandates “AI validation panels” for medical tools, ensuring human oversight at every stage.
The Future: Where Do We Go From Here?
By 2030, experts predict AI-Driven Scientific Discovery will:
- Cut average drug development costs by 65%
- Enable real-time climate adaptation planning via digital twins
- Unlock nuclear fusion feasibility through plasma behavior modeling
But as Dr. Joy Buolamwini, founder of the Algorithmic Justice League, reminds us:
“Technology mirrors its creators. If we want AI to serve all humanity, we need all humanity represented in its creation.”
This vision aligns with why AI in space exploration pushes boundaries while demanding inclusivity.
Why Diversity Shapes AI’s Future
Homogenous AI development risks blind spots, as seen in biased medical models. AI in research breakthroughs thrives when diverse voices shape algorithms, ensuring solutions serve all. The fearless call: tech must prioritize representation, or we’ll inherit a lopsided future.
Your Role in Shaping the Future
The age of AI-driven scientific Discovery isn’t just for researchers—it’s for policymakers, ethicists, and engaged citizens. Subscribe to our newsletter for monthly deep dives on ethical AI in science, open-source tools, and breakthroughs that matter.
For more on AI’s transformative potential, explore how AI in mental health detection is saving lives through early intervention.