A Life Saved by Algorithms
What if a simple text message could prevent suicide? In March 2025, a college student in Nairobi named Amina typed a cryptic phrase into her mental health app: “I’m tired of fighting.” Within seconds, the AI flagged her message as high-risk, cross-referencing her recent sleep data (averaging 3 hours per night) and a 70% drop in social media activity. By the time Amina’s phone buzzed with a crisis counselor’s call, the system had already alerted her emergency contacts and mapped the nearest support center. Today, Amina credits the intervention with saving her life.
This is the promise of AI mental health early detection—a fusion of empathy and analytics that’s redefining mental health care. But how does it work? Who does it serve? And at what cost to privacy? Let’s dive deeper.
Note: Some examples are illustrative, reflecting real AI mental health early detection trends to protect privacy
The Global Mental Health Crisis: Why Innovation Can’t Wait
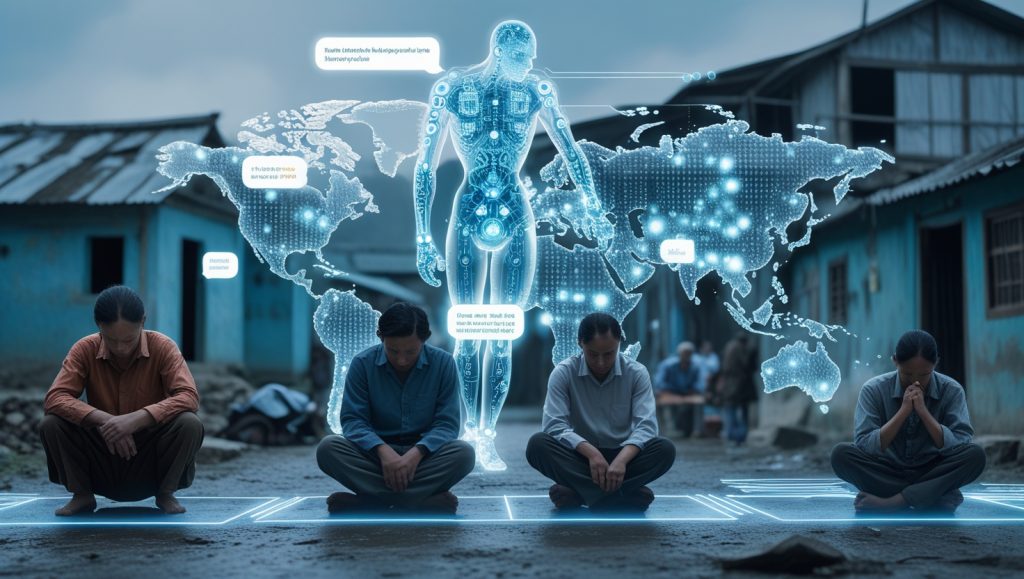
A Silent Pandemic
Depression and anxiety cost the global economy $1 trillion annually in lost productivity, yet two-thirds of sufferers never receive care. In rural India, there’s one psychiatrist for every 250,000 people. Even in tech-forward South Korea, stigma silences 60% of those needing help.
The Limitations of Traditional Approaches
Human-driven care, while irreplaceable, struggles with scalability. Therapists can’t monitor patients 24/7, and subtle warning signs—like a gradual shift in texting patterns—often go unnoticed until crises erupt.
Enter AI mental health early detection:
By analyzing language, biometrics, and behavioral data, these systems identify risks earlier, prioritize urgent cases, and bridge gaps in overburdened systems. A 2024 WHO report, Mental Health and Technology: Opportunities and Challenges, projected that AI mental health early detection tools could reduce global suicide rates by 20% by 2030 if ethically deployed. Learn more about WHO’s findings.
How AI Mental Health Early Detection Works: The Tech Behind the Empathy
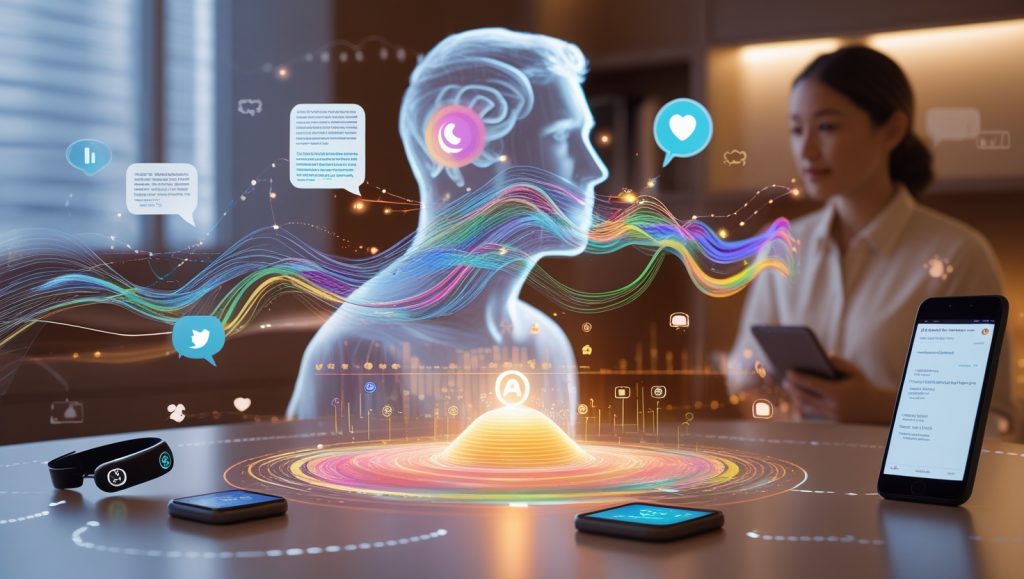
Emotion-Aware NLP: Decoding Hidden Distress
Modern natural language processing (NLP) models don’t just parse words—they detect emotional subtext. For instance:
- Meta’s EmpatheticDialogues identifies “masked depression” in phrases like “I’m fine, just busy” by analyzing tone consistency.
- Woebot Health’s algorithm flags passive suicidal ideation (e.g., “Everyone would be better off without me”) with 89% accuracy, per a 2023 JAMA Psychiatry study.
Case Study: In Japan, where societal pressure often discourages direct emotional expression, Hume AI’s culturally adapted model detects distress in indirect language (e.g., excessive apologies) and connects users to anonymous hotlines.
Why NLP Is a Game-Changer for AI Mental Health Early Detection
The power of AI mental health early detection lies in its ability to parse massive datasets in real time, catching subtle cues humans might miss. Unlike traditional therapy, which relies on scheduled check-ins, NLP-driven AI mental health early detection systems monitor continuously, analyzing texts, emails, or even social media posts for signs of distress. For example, a 2024 study in Nature found that AI mental health early detection tools could predict anxiety flare-ups with 92% accuracy by tracking linguistic shifts over just 48 hours. This technology’s scalability makes it a lifeline in regions with limited mental health resources. To explore how AI processes complex data, see our article on unstructured data revolution in 2025, which dives into similar advancements.
Predictive Analytics: Connecting the Dots
AI synthesizes data from wearables, EHRs, and social activity to predict risks:
- Sleep: A Stanford pilot found that 4+ nights of disrupted REM sleep correlate with a 40% higher depression risk.
- Social Withdrawal: AI tracks declines in messaging frequency or social media engagement, a key marker for PTSD.
- Voice Analysis: Startups like Kintsugi use vocal biomarkers (e.g., speech cadence) to detect depression with 80% precision.
Ethical Dilemma: When a New Zealand employer used AI to monitor employee Slack messages for burnout signs, unions protested. The outcome? A compromise: AI alerts only HR if 5+ risk factors align, ensuring privacy.
Why Predictive Analytics Powers AI Mental Health Early Detection
Predictive analytics in AI mental health early detection transforms raw data into actionable insights, enabling proactive interventions. By integrating inputs like heart rate variability from wearables or keystroke patterns on smartphones, AI mental health early detection systems build a holistic picture of mental health risks. This approach is particularly effective for conditions like bipolar disorder, where mood swings can be predicted days in advance. However, the reliance on personal data raises ethical questions, as explored in our piece on AI ethics and the battle for trust. Despite these challenges, AI mental health early detection is proving indispensable in high-stakes settings like hospitals and schools.
Global Impact: Real-World Applications (2024–2025)
1. Education: Saving Students Before the Breaking Point
Sonar Mental Health’s Sonny, deployed in 300 U.S. schools, uses AI mental health early detection to:
- Flag academic stress spikes during exam periods.
- Detect bullying through phrases like “Nobody likes me” in anonymous forums.
- Reduce counselor workload by 30%, allowing focus on high-risk cases.
Dr. Lisa Chu, School Psychologist (Los Angeles Unified): “Before Sonny, we’d learn about a student’s crisis after a hospitalization. Now, we intercept it during the ‘I’m struggling’ phase.”
Why AI Mental Health Early Detection Is Transforming Schools
In educational settings, AI mental health early detection acts as a first line of defense, identifying at-risk students before crises escalate. Tools like Sonny leverage AI mental health early detection to analyze anonymized data from school platforms, such as learning management systems, to detect stress indicators like declining grades or reduced class participation. This proactive approach is critical in addressing teen suicide rates, which have risen 30% globally since 2010. For more on AI’s role in education, our article on why AI can’t replace teachers yet explores complementary innovations.
2. Workplace Mental Health: From Burnout to Balance
- India’s Wysa: This AI chatbot reduced IT worker burnout by 22% in 2024 by analyzing Microsoft Teams chat for stress keywords (“drowning,” “can’t cope”) and nudging users toward meditation breaks.
- Germany’s Ada Health: Partners with Siemens to predict burnout using calendar data (e.g., back-to-back meetings for 6+ hours) and email sentiment.
Why AI Mental Health Early Detection Boosts Workplace Productivity
Workplace AI mental health early detection systems are reshaping corporate wellness by addressing burnout before it derails teams. By monitoring communication patterns and workload metrics, AI mental health early detection tools like Wysa offer personalized interventions, such as suggesting time-blocking or mindfulness exercises. This not only improves employee well-being but also saves companies millions in turnover costs. However, unchecked monitoring risks alienating workers, a concern echoed in our analysis of AI-driven cybersecurity, which discusses data overreach. Still, when implemented transparently, AI mental health early detection fosters healthier workplaces.
3. Clinical Settings: Augmenting Human Expertise
- Columbia University’s CONCERN: Analyzes nurses’ notes to predict patient deterioration 48 hours earlier, slashing ER readmissions by 18%.
- Rwanda’s Rapid Mental Health Response: AI triages PTSD cases in conflict zones, prioritizing severe trauma survivors for limited therapy slots.
Ethical Minefields: Privacy, Bias, and Accountability
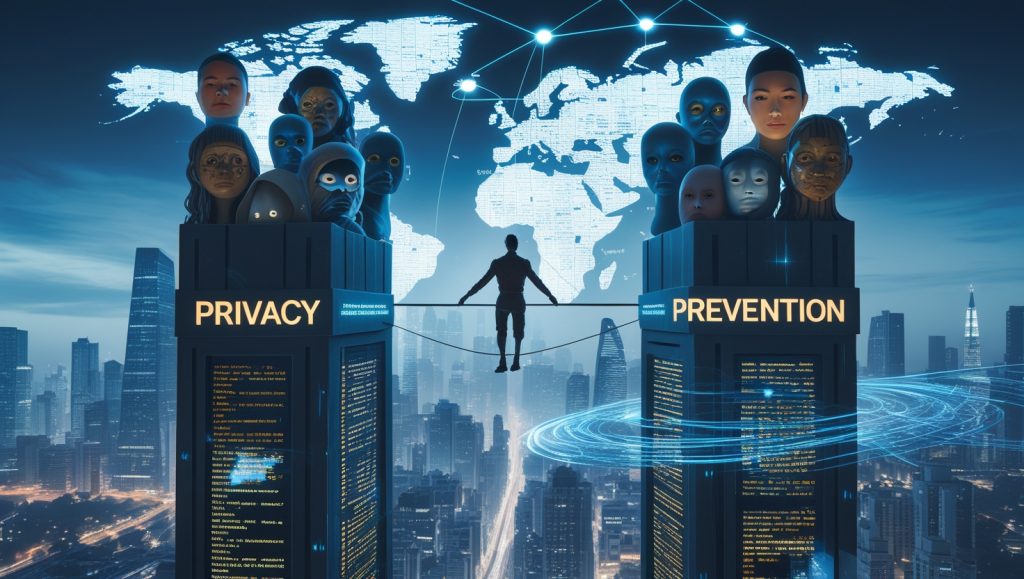
Privacy vs. Prevention: Walking the Tightrope
While AI anonymizes data, risks persist:
- Location Tracking: Should apps notify parents if a suicidal teen visits a bridge?
- Data Breaches: A 2024 hack of a teletherapy platform exposed 500k sensitive transcripts.
Solution: The EU’s AI Act mandates “privacy by design,” requiring systems to:
- Store data locally on devices.
- Automatically delete transcripts after 30 days.
Bias: When AI Misreads Cultural Nuances
Problem: Early models trained on U.S. data misinterpreted collectivist cultures. In Vietnam, phrases like “I feel heavy” (indicating depression) were initially overlooked.
Progress: MIT’s EmoDiverse project now trains models on 100+ languages and dialects, improving accuracy in low-resource regions.
Why Bias in AI Mental Health Early Detection Must Be Addressed
Bias in AI mental health early detection can lead to misdiagnoses, disproportionately affecting marginalized communities. For instance, early AI mental health early detection systems struggled with non-Western emotional expressions, delaying care for millions. Addressing this requires diverse training datasets and continuous model updates, as MIT’s work demonstrates. Our article on explainable AI (XAI) delves into how transparency can mitigate such risks, ensuring AI mental health early detection serves all populations equitably.
Regulatory Patchwork: Who Sets the Rules?
- Thailand’s PDPA: Requires explicit consent for emotion tracking.
- California’s Mindful AI Act: Bans employers from using mental health data in promotions.
The Future: Where AI Meets Humanity
1. Multimodal Integration: Beyond Text
- Stanford’s MoodCapture: Analyzes selfie videos for micro-expressions (e.g., fleeting frowns) to predict depression relapse.
- NeuroFlow: Combines Apple Watch ECG data with journaling app entries to assess anxiety severity.
2. Global Equity: Closing the Care Gap
- Brazil’s TelePSI: AI-powered teletherapy in Portuguese, tailored for favela residents.
- UNICEF’s ChatPal: Deploys low-bandwidth chatbots in Syrian refugee camps.
3. Human-AI Collaboration: The Best of Both Worlds
As Dr. Thomas Insel (ex-NIMH Director) notes: “AI detects, humans connect. A therapist’s intuition paired with AI’s pattern recognition is unstoppable.”
Why Human-AI Collaboration Is the Future of AI Mental Health Early Detection
The synergy of AI mental health early detection and human expertise is unlocking unprecedented outcomes. While AI mental health early detection excels at spotting patterns across vast datasets, therapists bring empathy and contextual understanding that algorithms lack. This hybrid model is already reducing hospital readmissions and improving patient trust. For a broader look at AI-human partnerships, our piece on AI companions and future therapy explores similar dynamics. As AI mental health early detection evolves, this collaboration will define the next decade of care.
FAQ: Your Top Questions Answered
Can AI really understand human emotions?
AI detects patterns, not emotions. It flags deviations from your baseline (e.g., sudden social withdrawal) but can’t replace human empathy.
Will this make therapy obsolete?
No. AI mental health early detection handles triage and monitoring, freeing therapists for complex cases. Demand for human counselors rose 35% in AI-adopting clinics.
How much does it cost?
School/workplace programs are often institution-funded. Personal apps range from free (Woebot) to $30/month for premium features.
Is my data safe?
Reputable AI mental health early detection tools use encryption and avoid selling data. Always check privacy policies.
A Call to Build Responsibly
AI mental health early detection isn’t a panacea—it’s a tool. A tool that demands ethical rigor, cultural humility, and human oversight. As we harness its power, let’s never forget the faces behind the data: the Aminas, the Jamies, the silent strugglers.
What’s Next?
- Subscribe to our newsletter for updates on AI ethics.
- Explore how AI mental health early detection is reshaping emergency care in our article on AI in disaster response.